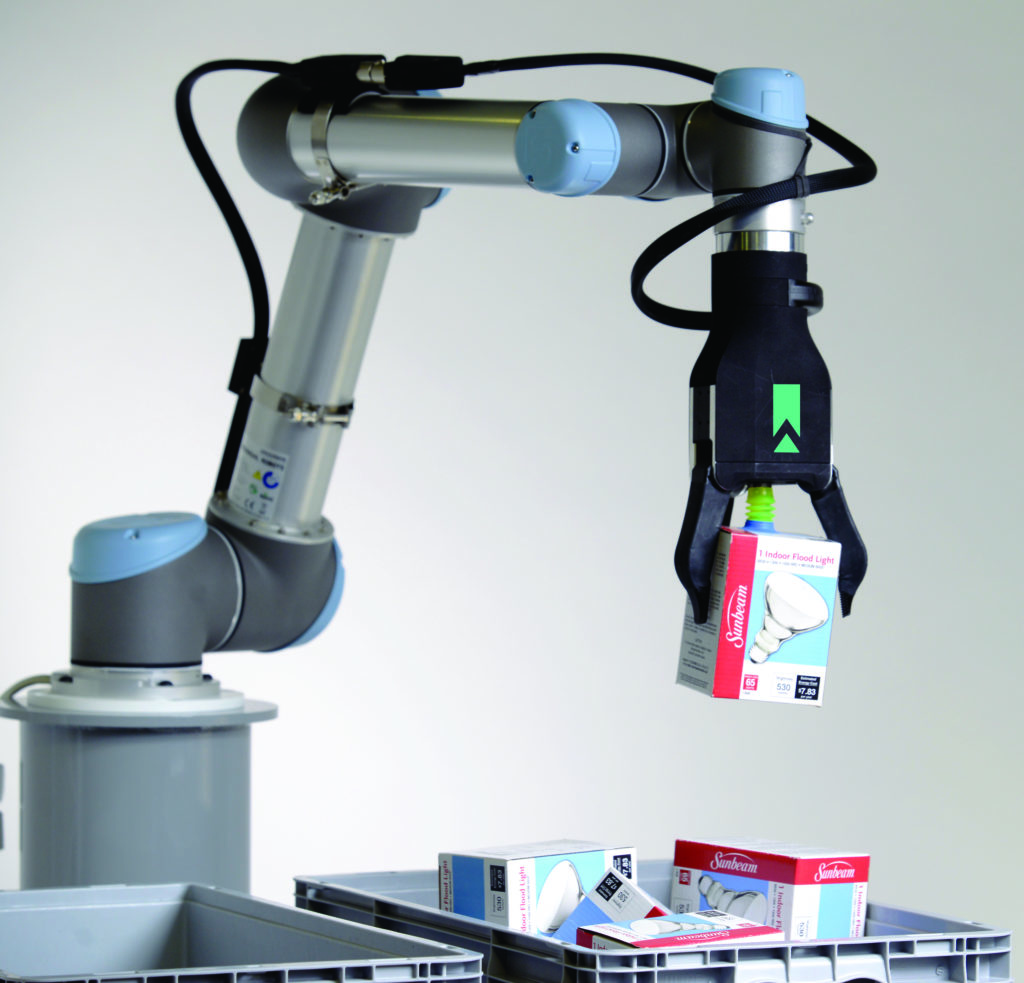
Robots are becoming more sophisticated for grasp operations. Source: RightHand Robotics
You sign into your workstation. A tote of mixed items arrives in front of you. You reach in, pick an item out, and place it in a container on the conveyor to your side. This incredibly simple task, done in a matter of seconds and without much thought, illustrates what a highly evolved machine you are.
Robots traditionally have struggled with this type of work. It’s an unstructured environment. The mix of items is not predetermined. The relative position and orientation of items are not fixed. The tote’s position on arrival may be reasonably consistent, but not perfect. The outbound container may be one of several queued up. There also may be other items in the space that you need to avoid.
How are autonomous robotic systems performing on simple pick-and-place tasks in similar real-world scenarios?
Degrees of autonomy
It’s helpful to use some formalism to assess degrees of autonomous activity for such systems. Thankfully, the Society of Automotive Engineers has published a recommended practice for autonomous vehicles that we can borrow from for the sake of this discussion.
Commercial picking systems today range from Level 1 to Level 4 autonomy. At the lower end of the scale, human-in-the-loop offerings can have reasonable range and reliability, but struggle to maintain high rate. Keep in mind that a pick cycle can be from 3 to 9 seconds, depending on the degree of complexity of process steps (barcode scan or not, for example) and reach requirements. There’s not much time for a remote human tele-operator to make decisions and control several systems simultaneously.
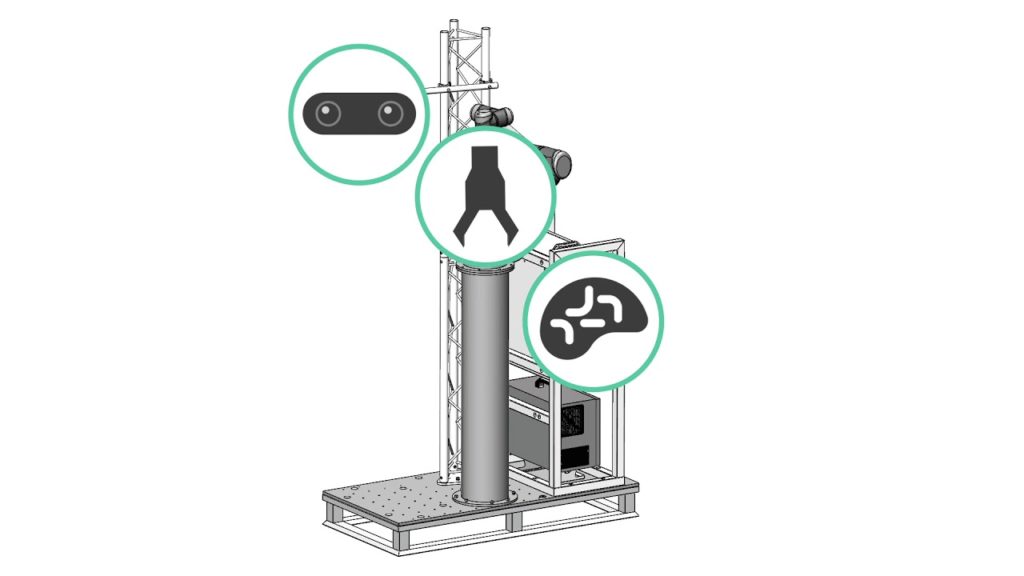
Source: RightHand Robotics
At the higher end of the scale, Level 4 systems have run continuously during peak-season volume with limited human intervention and at high rate, comparable with trained people doing similar tasks. These systems work within a bounded space and range of possibilities, including variability in incoming items and congestion on downstream equipment.
They benefit from integration with other automation systems in the facility to help clear fault conditions and manage a range of exception cases. But the robot is never required to react to issues arising from non-adjacent workflows or other areas in the warehouse, that might be expected for Level 5.
For robotic warehouse applications, where the objective is to multiply human productivity by having one person minimally involved in managing the work of a fleet of robots, the corresponding automation required is Level 4, “High Automation.”
Gripper design and OEE
Warehouse operators using automation strive for high overall equipment effectiveness (OEE). OEE is a measure of the overall throughput of the equipment, factoring in utilization and quality or error-free success rate. For a robotic picking system used in warehouse applications, the important performance parameters are as follows:
- The range, or variety of items that can be handled, which drives utilization
- The robot machine rate, which is how many units per hour the system processes and relates to throughput
- Reliability, which includes quality — no missed picks, accidental multiple item picks, dropped items or damaged items, or “M2D2” error metric — autonomy (Level 4), and availability
While a number of factors contribute to how a robotic picking system delivers these 3Rs (range, rate, and reliability) of performance, many are linked to the grasping approach itself.
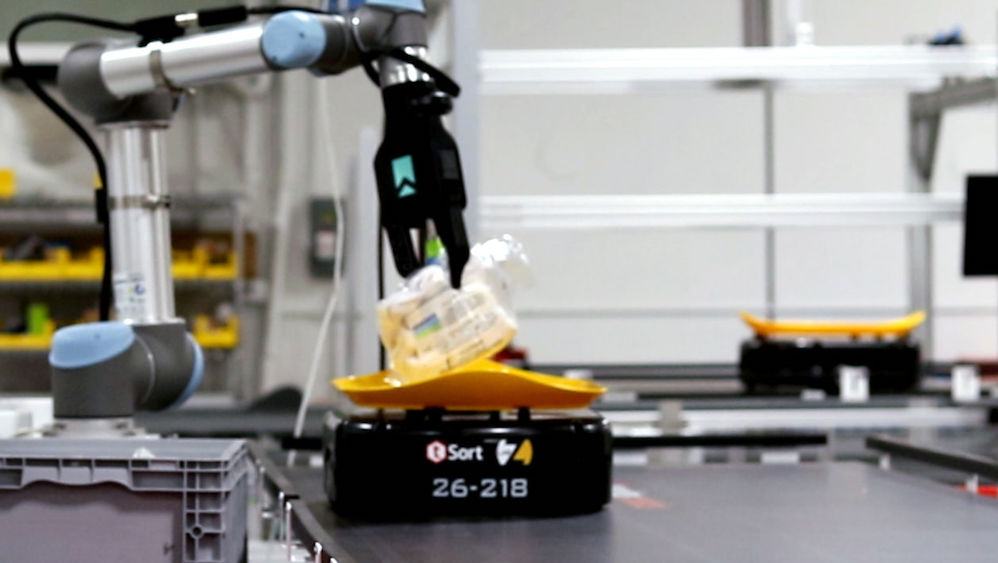
RightHand Robotics’ RightPick piece-picking system uses cameras, machine learning, and a robotic arm with a gripper to grasp objects. (Source: RightHand Robotics)
One design goal is to provide versatility in dealing with item range, including item slotting (e.g. source tote subdivisions). Considering an assortment of everyday products, there is a requirement to handle items up to 2 kg. The gripper needs a large enough hand span to grasp these items while still fitting into narrow compartments.
There is a need both for the lifting strength of a suction array, while still being able to attach a single cup to a small object. Combining a suction ‘stalk’ in tandem with gripper fingers enables the system to reach into tight spaces, singulate or grasp an object from the mix of items via suction, then gently grab the object to ensure stability during transport.
One of the largest reliability challenges for robotic pick-and-place tasks is the small size and relative fragility of the end-effector mechanisms involved. A robotic gripper that is too complicated will easily break if an unanticipated collision occurs. This could happen, for example, if a conveyor system leaves some uncertainty in a tote’s position when presenting it to the robot.
Compliant, underactuated fingers can be designed to achieve the grasp performance of a highly articulated robot hand with many fewer actuators or machine elements. Such fingers, manufactured from durable rubber materials, can withstand impacts from any direction and still continue to function, and they will last for millions of cycles.
Revisiting item storage practices to optimize pick performance
Even with thoughtfully designed grippers, early adopters of robotic picking systems are realizing that there is value to revising storage policies, removing excess packaging and trash, and modifying packaging to make items more robot grasp-friendly.
For example, storing bagged sweatshirts in a bin with narrow edge of the packages standing up and filling the bin as tight as possible, makes it difficult to make the initial pick. These items could be placed flat to make picking easier.
Loose packaging materials and other trash in a tote can be picked instead of the intended product, depending on the capabilities of the vision system to distinguish one from the other. And loose or torn packaging can come apart while the item is being lifted, leaving the product behind in the source bin. Minimizing these issues as part of new standard inbound product-handling processes enhances the autonomous performance of the system.
Drivers wanted for robotic grasp
These factors highlight the interaction between the computer vision system, the smart gripper, and the artificial intelligence software control systems and deep learning processes — what we think of as integrated hand, eye, coordination — and the role of the people managing and operating a robotic fleet in the context of an overall plan for bringing autonomous picking online. The former provides Level 4 autonomy and 3R performance, the latter uses these capabilities to achieve high OEE that delivers value to the business.
Autonomous robotic picking solutions continue to mature and gain traction in the marketplace. There’s a lot of cool tech, including the grippers themselves. Operators that master “driving” their robot fleet can further elevate the performance level of the machines, gaining a competitive edge in the race to provide world-class order fulfillment.
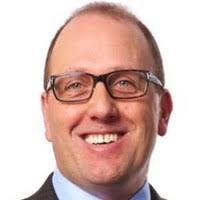
Vince Martinelli, RightHand Robotics
About the author:
Vince Martinelli is head of product and marketing at RightHand Robotics, a leading provider of robotic piece-picking solutions for e-commerce order fulfillment and intralogistics.
Tell Us What You Think!