The first step to a big data management and analytics strategy is determining what you want to do with it, said speakers at a workshop before RoboBusiness 2018.
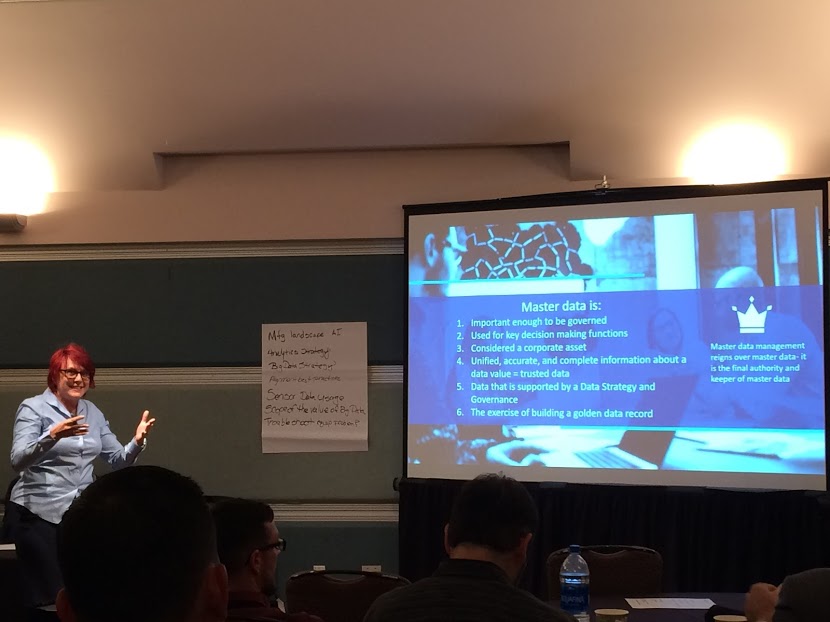
SANTA CLARA, Calif. — Like collaborative robots and artificial intelligence, big data has gotten its share of buzz from consultants and the high-tech media. Thanks to cheaper sensors, more autonomous robots, and improving analytics tools, manufacturers are expecting actionable insights. So what data management lessons can organizations draw from in devising their data strategies?
The half-day workshop on “Planning and Implementing a Successful Data Management Strategy” here yesterday included speaker experiences, attendee input, and data management examples. The panelists were Ed O’Brien, a principal at Competitive Directions and a Robotics Business Review contributor; Allen Thompson, director of knowledge management and analytics at Oneida Nation Enterprises; and Kim Kaluba, senior product marketing manager, data management, at SAS.
Attendees of the packed workshop included executives from manufacturers of all sizes, robotics suppliers, and government-funded research institutions. Vertical markets represented included aerospace, healthcare, energy, custom manufacturing, and supply chain.
Here are some data management lessons from the pre-RoboBusiness event:
1. Know your business goals before you plan
Before your organization jumps into data analytics, a data strategy, or even an AI project, figure out what you want to do with the data, explained Kaluba.
Answer the question, “Why are we doing this?” Thompson said.
“A fundamental component is to align people, processes, and technologies,” Kaluba said. “It sounds really simple, but lots of organizations forget what they’re trying to do. You need to step back and have those initial conversations.”
O’Brien observed that two-thirds of corporate data is still in spreadsheets or databases that are siloed and not yet subject to data management practices.
“Thirty-three percent of C-Suite believe their organization’s data is inaccurate, and 77% of data users can’t access all relevant data they need,” he said, referring to an Experian study. “Seventy-two percent of organizations say data quality impacts their business.”

Allen Thompson has worked on data, analytic, and intelligent solutions for casinos, banks, and retail operations.
Thompson noted that many businesses collect transactional and structured data with the hope that it might be useful, but with no idea on what problems data analysis could help solve.
“Up to 75% of data is unstructured, which is more complicated,” he said. “That’s why the concepts of a data dictionary and lifecycle management are important.”
Kaluba asserted that a big data strategy should be “tightly coupled” with data management and analytics. She explained that they’re important because they do the following things:
- Ensure that data can be used, shared, and moved easily and efficiently.
- Data is viewed and managed as a corporate asset. “It needs to get the love it deserves from those at the C-level,” Kaluba said.
- Establish common methods, priorities, and processes to manage and share data in a repeatable manner.
The components of a data strategy, according to SAS, are identifying the data that’s collected, storing it, provisioning it as needed, integrating it into enterprise systems, and governing it.
An attendee from a leading test equipment manufacturer pointed out that the benefits of applying big data from the Industrial Internet of Things (IIoT) to deep learning might not immediately transform a business. Instead, he said, predictive maintenance and other applications could drive efficiency savings.
2. Build a strong internal data management team
Most companies don’t document what their goals are for a data strategy, Kaluba said.
“You need time, money, and patience,” said Thompson. “You also need to deal with internal fiefdoms.”
“People think, ‘Let’s hire a data scientist or use AI,’” he added. “Companies tend to buy software, which is seemingly easier than dealing with process problems or people.”
“Should a chief robotics officer or chief data officer be created?” asked O’Brien.
“Not many companies have figured it all out,” replied Thompson. “It’s not just titles; you need everybody at the same page.”
He recalled that at the bank at which he once worked, there were 8,200 metrics and 11,000 reports rather than a manageable handful.
“It was sometimes easier to build a new report,” Thompson said. “You can quickly be overwhelmed, but you can’t run a business without marketing, finance, and other data.”
In response to a question, Thompson said that it’s possible to start at the departmental level, since “trying to build consensus at a global organization sometimes just don’t get off the ground.”
“Sometimes it’s hard to turn the corporate ship,” Kaluba said. She described the role of “data stewards,” representing different lines of business and responsibility.
An attendee from a medical device maker said that in his organization, each unit in a value stream has ownership of its data.
3. Big data management and governance
A big problem is an overload of data, with “no single version of the truth,” said the panelists. Companies can have a good data management strategy, but then an acquisition could result in parallel projects and data.
“Big data management requires cultures to change,” Kaluba said. “Data is often viewed as a byproduct than as a corporate asset.”

Kim Kaluba talks about the importance of master data and data governance.
Kaluba said that a major home improvement retailer wanted a single view of customer data to support engagement efforts. It bought a master data management (MDM) system, but after five years, $200 million, and two CMOs, it still doesn’t have a view.
“Master data management data is important enough to be governed, since it’s used for key decision-making functions,” she said. “It must be unified, accurate, and complete to be trusted.”
“Like the iterations in the movie Multiplicity, after two years, copies become less smart,” said Thompson. “Deduping is critical to avoiding multiple versions of the truth.”
Security should be part of data governance, Thompson said. He said his bank realized that the hard way after a zip drive was used to steal credit-card data.
“Fortunately, big data and analytics tools are increasingly available to manufacturers,” Kaluba noted, citing Microsoft, MicroStrategy, Tableau, SAP, SAS, and Qlick.
4. Start small for big wins later
“You need small, quick wins to get executive support while building toward longer-term goals,” said Thompson. “I would have meetings every three weeks to keep that contact going.”
Condition monitoring, IT asset management, managing robots, and enforcement of service-level agreements are examples of why big data is important in manufacturing, Kaluba said. She shared a number of other brief case studies in telecommunications, environmental science, and midsize and multinational manufacturing.
Industry 4.0 initiatives require lots of data, said Thompson. From supply chain management and robotic process automation to augmented reality and virtual reality, the applications are still growing, he said.
“In the past five years, U.S. retail supply chain operations that have adopted data and analytics solutions have seen increases of operating margins approaching 20%,” said O’Brien, citing a McKinsey study.
However, the utilization of data and analytics has been uneven, with the retail industry capturing only 30% to 40% of potential value, he said. Manufacturing has captured only 20% to 30% of potential value.
5. Big data isn’t a cure-all
“A lot of companies fall into the trap of data hoarding,” Thompson said. “The process of collecting the data continuously can be expensive. You need to articulate day-to-day data mining versus finding innovative insights.”
An attendee asked why companies haven’t realized the value of big data management for Industry 4.0.
“We’re a little ahead of the curve — sensors and data are evolving,” Kaluba said. “In addition to data scientists, we’re seeing a new title: ‘data engineers,’ who prepare data for analytics and engineers.”
“Siloed data is still a challenge,” said O’Brien. “Robotics companies will send their own technicians on site before sharing that information with the manufacturer using the robots.”
The speakers agreed that not every business problem requires big data and that the best companies are those where everyone is on the same page.
Note: Editor Keith Shaw contributed to this article.